Drivers of water quality in Afromontane-savanna rivers
- 1Department of Fisheries and Aquatic Science, University of Eldoret, Eldoret, Kenya
- 2Department of Water Resources and Ecosystems, IHE Delft Institute for Water Education, Delft, Netherlands
- 3Department of Ecology, University of Innsbruck, Innsbruck, Austria
Although several studies have investigated the relationships between water quality in rivers and the types of land use within their catchments, many aspects of these relationships remain unclear in Afromontane-savanna rivers, especially the interactions between catchment land use, seasonality and stream size. Afromontane-savanna catchments present a unique situation where headwater regions and lowlands have experienced more dramatic land cover change, but mid-elevation regions remained rather natural. We examined the influence of seasonality, catchment land use and stream size, including their interactions, on water physico-chemistry, nutrients and major ions in the Afromontane-savanna Mara River in Kenya, using data collected from 2010 to 2018 at >150 sampling sites in the Kenyan part of the river. We developed generalized linear mixed models (GLMMs) to explore the influence of seasonality (dry and wet seasons), land use (forest, mixed, agriculture and grasslands), stream size (stream orders 1–7), and their interactions on river water quality. Water quality variables included physico-chemical measures (pH, dissolved oxygen [DO] concentration, temperature, electrical conductivity, total dissolved solids [TDS], turbidity, total suspended solids [TSS] and particulate organic matter [POM]), nutrients (
Introduction
Streams and rivers are sentinels of conditions in the catchments they drain (Karr, 1998; Williamson et al., 2008). Because of the strong influence of catchments on rivers (Hynes, 1975), many studies have sought detailed analysis of water quality to infer biogeochemical and hydrological processes at the catchment scale. Catchment influences on the condition of rivers are assumed to be strongest at the headwaters where the linkage between the terrestrial and the fluvial domain is strongest (Vannote et al., 1980; Lambert et al., 2011). Yet, the contribution of catchments and headwater streams is important to the physical and biogeochemical characteristics of entire river basins, especially in terms of the transfer and transformation of carbon, nitrogen, phosphorus, and associated elements (Cole et al., 2007; Battin et al., 2009; Aufdenkampe et al., 2011).
Land use change from natural vegetation to other uses (e.g., agriculture, urbanization, or grazing) is one of the major causes of water quality degradation and loss of ecological integrity in rivers worldwide (Allan, 2004; Dudgeon, 2010; Vörösmarty et al., 2010; Brauns et al., 2022). Catchment land use change influence the structure and functioning of rivers mainly through changes in runoff and erosional processes, organic matter dynamics, the input of nutrients and major ions, in-stream ecosystem processes such as primary production, organic matter processing and nutrient cycling, and the composition of biological communities (Dudgeon et al., 2006; Bernot et al., 2010; Petrone, 2010; Tank et al., 2010; Woodward et al., 2012; Fugère et al., 2018). The proportion of different land use types in a catchment is closely correlated with many water quality parameters in aquatic systems (Lenat and Crawford, 1994; Tong and Chen, 2002; Hwang et al., 2016). For instance, there is agreement that catchments with high proportions of developed land area (i.e., urban land use) tend to have higher concentrations of water pollutants and nutrients (Roberts and Prince, 2010; Nagy et al., 2012; Hwang et al., 2016). Similarly, levels of electrical conductivity, major ions and suspended sediments in rivers increase with the proportion of agricultural land (Minaya et al., 2013; Kilonzo et al., 2014; Masese et al., 2017). However, some studies have shown that the influence of land use on some water quality parameters in rivers does not only depend on the proportion of different land use types in the catchment area, but also on the size and use of the riparian zone (Minaya et al., 2013; Fierro et al., 2017; de Mello et al., 2018; Hilary et al., 2021; Kadeka et al., 2021).
Apart from spatial variability, surface water quality also varies greatly over time or temporally (Chang, 2008; Guo et al., 2019, 2020). In this regard, seasonality, or the flow regime, which in the tropics is dominated by the difference in the amount of rainfall and runoff generated from the catchments (i.e., wet vs. dry season), can directly or indirectly influence many biogeochemical and ecological processes in rivers, including water quality. Pollutants entering a river system normally depend on rainfall patterns, which drive the rainfall-runoff processes that underpin constituent mobilization and transport (Lintern et al., 2018). During the wet season, increased runoff from farmlands and developed areas can increase the levels of sediments, nutrients and organic matter transported by rivers (Kilonzo et al., 2014), although for most constituents there is often a lag and hysteresis caused by a non-linear relationship between discharge and concentration (Sanderman et al., 2009; Lambert et al., 2011; Bouillon et al., 2012). In intermittent or ephemeral rivers, cessation of flow creates a series of isolated pools that may or may not be connected through interstitial flow. Thus, the spatial and seasonal shifts in flow levels can interact with land use and other human activities to influence the composition and concentrations of materials transported by rivers (Paetzold et al., 2008; Roach et al., 2014; Rodrigues et al., 2018). Therefore, it is crucial to understand patterns and changes in average water quality across broad spatial and temporal scales to develop sustainable management strategies (Thompson et al., 2011; Godsey et al., 2019).
Studies on the spatial and temporal dynamics and determinants of water quality in Afromontane-savanna river systems are very limited. Nevertheless, water quality changes in these systems can occur at short spatial and temporal scales because of the highly heterogenous nature of the landscapes created by rapid changes in elevation, amount of rainfall, geology and vegetation type. Usually, conditions in the uplands are characterized by higher amounts of rainfall and cooler temperature that support broadleaf tropical vegetation while in the lowlands the conditions are much drier, soils shallow and vegetation sparse and dominated by shrublands and grasslands (Tamooh et al., 2012; Abrantes et al., 2013; Aich et al., 2014; Mushi et al., 2019; Englmaier et al., 2020). Unlike other biomes, Afromontane-savanna rivers also present a unique situation where land use features vary both altitudinally and longitudinally from the headwaters. Specifically, while rivers are postulated to exhibit a prominent shift in physical and chemical factors from headwaters to the lower reaches (e.g., water temperature, different fractions of organic matter and their sources (Vannote et al., 1980; Downing et al., 2012; Creed et al., 2015), different patterns have been reported in African savanna rivers (e.g., Masese and McClain, 2012; Masese et al., 2015, 2022). In these landscapes, the abundance and biomass of large mammalian herbivores (both wildlife and livestock) also present a natural gradient characterized by low numbers in the forested uplands and high densities in the savanna grasslands in the lowlands. Rivers that drain these landscapes display a tight coupling with terrestrial ecosystems through the vectoring role of both livestock and large wildlife in transferring large amounts of organic matter and nutrients through egestion and excretion at watering and crossing points along the rivers (Subalusky et al., 2015; Iteba et al., 2021). African savanna landscapes are also emerging as new frontiers of land use change as human populations and their livestock migrate into marginal lands in semi-arid and arid areas to ease pressure on more productive and densely populated humid lands. As a result, the humid headwater regions and drier savanna lowlands have experienced more dramatic landcover changes, but mid-elevation regions remained rather natural. Thus, the postulated upstream-downstream gradients (e.g., Vannote et al., 1980) may not apply to these systems. Moreover, rivers draining savanna landscapes are seasonal with highly variable flow regimes characterized by cessation of flow during the dry season and flash flooding during the rainy season (McClain et al., 2014). These attributes of Afromontane-savanna rivers present intriguing scenarios for understanding the interactions among different drivers of water quality in rivers.
In this study, we investigated the influence of seasonality, land use and stream size/order on several water quality variables (physico-chemical measures, nutrients, and major ions) in Afromontane-savanna rivers using the Mara River, Kenya, as a case study. Our key objectives were to 1) identify the spatial variability in mean water quality variables at the river basin scale and across different land use types and stream sizes/orders; 2) determine the influence of seasonality, land use and stream size and their interactions as drivers of water quality in the river; and 3) determine the influence of stream size/orders on longitudinal trends in water quality variables in the river. We hypothesized that is be strong variability in both mean concentrations and water chemistry across land use types and between seasons, which would influence the role of stream size as a predictor of water quality in the river. The Mara River basin (MRB) is representative of many river basins in Africa that partially drain humid uplands and semi-arid lowlands with wide variations in elevation, rainfall and geology. Therefore, this analysis will contribute to understanding how water quality responds to land use change at the basin scale, which can be used to inform water resources management in the continent.
Materials and methods
Study area
This study was conducted on the Kenyan part of the Mara River basin (MRB, Figure 1). The Mara River is an Afromontane-savanna river that partly drains an Afromontane tropical forest and partly semi-arid grasslands and shrublands hosting large numbers of livestock and wildlife (Ogutu et al., 2016; Veldhuis et al., 2019). The headwaters of its two perennial tributaries, the Nyangores and Amala rivers, drain the larger Mau Forest Complex (MFC) in the Mau Escarpment, which is the most extensive tropical moist broadleaf forest in East Africa (Wass, 1995). In the middle and lower reaches, the Olale Orok, Sand and Talek tributaries drain the semi-arid grasslands and shrublands of the Maasai Mara National Reserve (MMNR) and adjoining conservancies in Kenya and the Serengeti National Park (SNP) in Tanzania (Figure 1).
In the highlands, the climate is relatively cool and seasonal due to the high altitude, and is characterized by distinct rainfall seasons and low ambient temperatures that fall below 10°C during the cold months of January-February. Rainfall varies with altitude with the highlands receiving around 2000 mm of rainfall per annum while the semi-arid lowlands in the MMNR and adjoining areas receiving around 1,000 mm. The altitudes in the basin range from 2,932 m around the sources in the Mau Escarpment to 1,134 m around Lake Victoria. Dry conditions are experienced during January-March and two wet conditions during March-July and October-November, which are periods for the long and short rains, respectively. However, variations sometimes occur at the onset and end of the rainy and dry periods. Potential evapotranspiration varies between 1,400 mm in the highlands to 1800 mm in the lowlands (Jackson and McCarter, 1994).
Until the middle of the past century, the MRB was covered by montane forest in the headwaters and a mixture of shrublands and grasslands throughout its middle and lower reaches in Kenya (Serneels et al., 2001; Mati et al., 2008). However, land use change to agriculture and grazing lands and settlements has reduced coverage of both the broadleaf forest in the headwaters and the grasslands in the middle reaches (Lovett and Wasser 1993; Obati, 2007). With human population growth in the area averaging 3% p. a., land use practices are bound to intensify with remnant forests and grasslands likely to be converted into farmlands, settlements and livestock grazing areas.
Study design
In this study, we used data on water quality variables that were collected from 2010 to 2018 covering both the wet season (April-June and October -December) and dry season (January-March and July-September). These data were collected from more than 150 sites spread out in the entire MRB in Kenya (Figure 1). Some of the data have been published in previous works, including Minaya et al. (2013), Masese et al. (2014a, b, 2017, 2022) and Iteba et al. (2021). Although data were available from 2010 to 2018, most of the data used were collected in 2010, 2012, 2016 and 2018.
To capture spatial and temporal variability in water quality across the entire river basin, sampling sites were in rivers draining a gradient of catchment land use from 100% forestry or grasslands to 100% agriculture. The sampling sites were then classified into four broad land use categories depending on the proportions of forest, grasslands, and agricultural land use in the entire catchment upstream of the sampling sites. Based on the Digital Elevation Model of Kenya (90 m by 90 m), obtained from the Shuttle Radar Topography Mission, catchments were delineated and the area of each major land use category upstream of each sampling site was calculated. Forest (FOR), grassland (GRAS) and agriculture (AGR) sites drained catchments with the proportion of catchment land use under forestry, grasslands, and agriculture >60%, respectively. Mixed (MIX) sites did not meet the catchment land use criteria for, GRAS and AGR sites, and had an intermediate mixture of two or three of the major land use types.
The forested (FOR) sampling sites were in Mau Forest Complex in the headwaters of the Amala and Nyangores rivers, which are the main and perennial tributaries of the Mara River. Along FOR streams, the riparian corridor was largely intact with a mixture of indigenous vegetation throughout the catchment (Figures 2A,B). Compared to AGR sites, water quality in FOR streams was less turbid even immediately after storm events (Figures 2B,C). The AGR and MIX sampling sites were in agricultural areas in the middle reaches of the MRB. While AGR sites drained catchments that were entirely on agricultural land, MIX sites were in catchments that were partly forested and partly agricultural, with none of the two land uses dominating with >60% (Figure 2D). MIX sampling sites were in agricultural areas (Figures 2E,F). People living in the adjoining areas of the MFC and MMNR in the AGR and MIX catchments are involved in semi-intensive subsistence agriculture of mainly tea, maize, beans and potatoes and animal husbandry. Along many agricultural streams on the highlands, indigenous riparian vegetation has been replaced by exotic Eucalyptus species and other exotic trees (Masese et al., 2014b; Kroese et al., 2020). Livestock footpaths that connect grazing areas to the rivers are also a common feature of many AGR and MIX catchments, and overused unpaved tracks are a major cause of erosion and surface runoff into the rivers (Kroese et al., 2020). Cattle entrance points to streams are generally highly disturbed and have degraded riverbanks, and the water is often turbid from livestock access and trampling even during the dry season (Figures I, J).
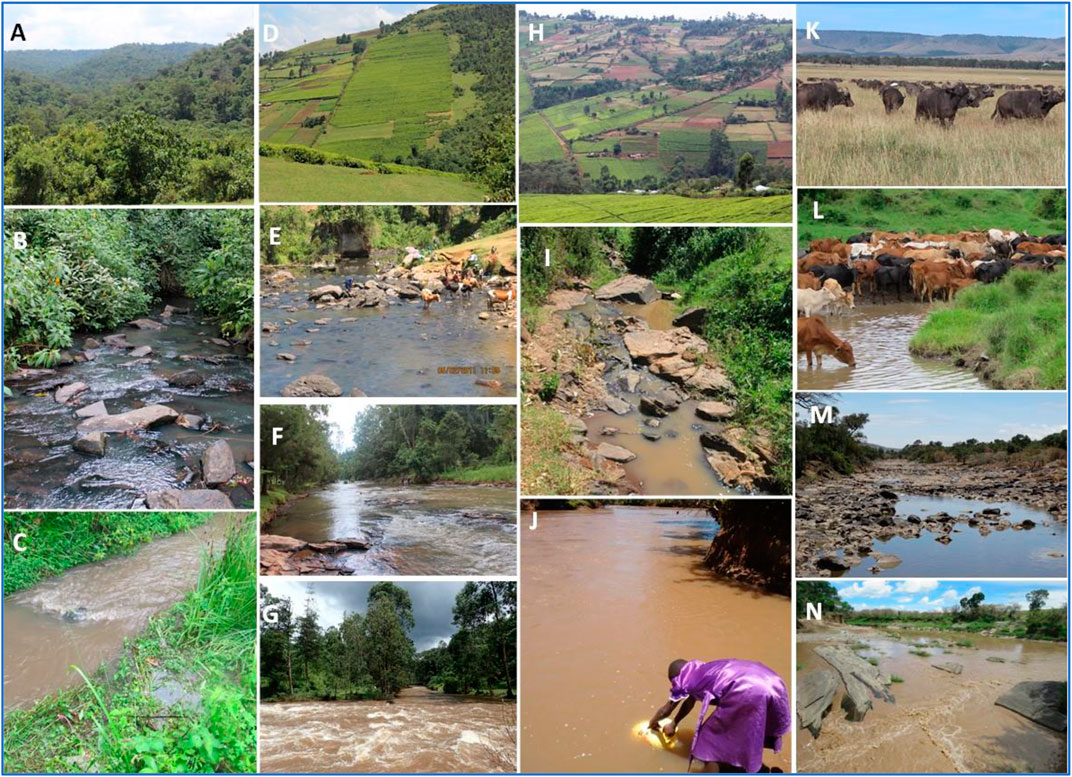
FIGURE 2. Sampling sites in rivers in different land uses in the Mara River basin, Kenya; Forest (A–C), Mixed (D–G), Agriculture (H–J) and Grasslands (K–N). Upper panel photos show the landscape in each of the four land uses (A) forested, (D) mixed land use, (H) agricultural land use, and (K) grasslands. The middle panel photos show the condition of streams in the different land uses during the dry season, (B) forested, (E,F) mixed land use, (I) agricultural land use, and (L,M) grasslands. The lower panel photos show the condition of the streams in the different land uses during the wet season, (C) forested, (G) mixed land use, (J) agricultural land use, and (N) grasslands. Photo credits: (A–H,N)–Frank Masese; (I,J)–Elizabeth Wanderi; (K,L)–Evans Ole Keshe; (M)–Paul Geemi.
The GRAS sampling sites were in the seasonal tributaries (the Olare-Orok, Talek and Sand rivers) draining semi-arid areas of the lower MRB basin (Figure 1). These tributaries drain undulating plains that are dominated by grasslands interspersed with shrublands (Figure 2K). Land use here is protected for wildlife in the MMNR and SNP, but the adjoining areas are used as conservancies for livestock grazing while at the same time allowing wildlife protection (Veldhuis et al., 2019). The Serengeti-Maasai Mara ecosystem is host to one of the largest populations of large wildlife in the world (Figure 2K), including >4,000 hippopotami (hippos) in the Mara River and seasonal tributaries in the MMNR and SNP (Kanga et al., 2011). This region hosts large herds of livestock which use the rivers for watering and crossing. Both large wildlife (mainly hippos) and livestock have a strong influence on water quality and ecological integrity of rivers through the input of large amounts of organic matter and nutrients through excretion and egestion (Subalusky et al., 2015; Iteba et al., 2021). Because of the large herds of livestock and the potential for overgrazing, there have been concerns about sheet and gulley erosion and sedimentation of rivers (Dunne, 1979; Dutton et al., 2018).
Water quality variables
The sampling methods and laboratory analysis of the water quality variables used in this study are presented in the Supplementary Materials. The data were divided into three categories or datasets: physico-chemical measures, nutrients and major ions. The data on physico-chemical measures included pH, dissolved oxygen (DO), temperature, electrical conductivity, total dissolved solids (TDS), turbidity, total suspended solids (TSS) and particulate organic matter (POM). The second dataset was on nutrients and included ammonium (NH4+), nitrates (
These water quality variables were considered in this study because of their exhaustive availability from other studies, where they have been proven to be responsive to catchment scale influences (e.g., agricultural, or urban land use) and local reach-scale disturbances such as wastewater disposal, clearance of riparian zones, livestock disturbance and other stressors (Minaya et al., 2013; Hwang et al., 2016; Iteba et al., 2021; Li et al., 2022). In many countries and monitoring programs, most of these water quality measures, such as dissolved oxygen (DO), pH, dissolved nitrogen and phosphorus, total nitrogen (TN) and total phosphorus (TP) form part of the criteria used for determining the condition of surface waters and for long-term monitoring and management of aquatic ecosystems (e.g., Hwang et al., 2016; Diamantini et al., 2018; Putri et al., 2018). All variables are expressed in mg/L except pH (−), electrical conductivity (μS cm−1) temperature = water temperature (°C). Concentrations below the detection limit were set to the detection limit.
Data analysis
We used principal component analysis (PCA) to reduce the dimensionality of the water quality data by collapsing the variables into individual PCs to avoid multicollinearity. The PCA also allowed us to identify correlated variables and the grouping of sampling sites based on land use and stream order. We included three PCs to describe water quality. The statistical differences in water quality variables between seasons, land use and stream sizes (including interactions), and the statistical significance of the PCAs was assessed using permutational analysis of variance (PERMANOVA) based on z-standardized data (McArdle and Anderson, 2001; Anderson and Walsh, 2013).
We used generalized linear mixed models (GLMMs) to determine the drivers of water quality variables as responses (Zuur et al., 2009; Pinheiro et al., 2017). Given our unbalanced study design (Figure 1), the use of GLMMs allowed us to account for temporal and spatial autocorrelation by including the year of sampling (Lamberti et al., 2010; Strauch, 2012; Chen and Lu, 2016; Wang et al., 2018) and sampling sites in the models. GLMMs were fitted using the lme function in the nlme package in the R platform (Pinheiro et al., 2017). For each response variable, GLMMs included season (dry and wet), land use (FOR, MIX, AGR, GRAS), stream order (order 1–7) and the proportion of agricultural land use/cover within the study reach’s drainage area (Percent AGR) as fixed effects, including potential interactions, and year and sampling site (nested within a year) as random effects. We included Percent AGR in the models because, in addition to the land use types, agriculture has been found in many studies to have a significant influence on water quality in rivers as an integrated measure of land-use influences on stream condition (Allan, 2004). In addition, Percent AGR was highly negatively correlated with the proportion of forested land use/cover, making it a good indicator of the effects of deforestation on water quality. As a continuous variable, Percent AGR was also better for fitting linear or non-linear relationships with water quality measures in the study area.
For comparison of the GLMM models, we fit an initial “full” model that included seasonality, land use, stream order and Percent AGR as fixed effects, and “year” and “site” as random effects. To identify the most parsimonious GLMM model that included only significant predictors for most water quality variables, we used a step-wise ANOVA approach based on the Akaike Information Criterion (AIC) to achieve an optimal model that explained most variation with the fewest predictors, with the lowest AIC among non-significant models indicating the best model (Burnham and Anderson, 2004). For each GLMM model, we computed marginal R2 (Rm2, variance explained by fixed factors) and conditional R2 (Rc2, variance explained by the entire model, i.e. by fixed and random factors) coefficients with the “r.squaredGLMM” function in the “MUMIN” package (Barton and Barton, 2015). To compare models, we used Akaike’s information criterion (AIC). The best-fit model based on AIC was identified using the AICcmodavg package (Mazerolle, 2017) in the R platform.
We used generalized additive models (GAMs) (Wood and Wood, 2015) to explore relationships in catchment characteristics and some select physico-chemical measures in the river. GAMs incorporate smooth functions that are more flexible in modelling nonlinear relationships (Hastie and Tibshirani, 1990). GAMs were fitted using the mgcv package (Wood and Wood, 2015) in the R platform (R Core Team, 2020) and built using penalized cubic regression splines with degrees of freedom automatically identified based on the generalized cross-validation score (GCV).
We used GAMs to explore relationships between the river distance from the source (RDS), stream order and the proportion of land under grasslands. We also used GAMs to relate in situ turbidity data (NTU) and particulate organic matter (POM, mg/L) to total suspended solids (TSS) concentration (mg/L). To explore the water quality variables in the study area further, we also evaluated the influence of stream size on longitudinal trends in water quality variables in the Mara River using GAMs. Instead of using stream order (Strahler, 1957), we used the continuous river distance from the source (RDS) as a measure of stream size (Rasmussen et al., 2009; Masese et al., 2015, 2022). For each sampling site, RDS was calculated as the square root of the drainage area in km2 (Rasmussen et al., 2009) as a general measure reflecting the linear dimension of a watershed. This measure of stream size is based on the understanding that the average length of stream paths leading to a point in the drainage can be expressed as a power function of the drainage area (Gregory and Walling, 1973) with the exponent 0.5 (Smart, 1972).
In all statistical analyses, response variables were log-transformed to meet model assumptions of normality when appropriate, and all analyses were conducted with an alpha of 0.05. Statistical analyses were performed with R version 4.0.2 (R Core Team, 2020) using the packages vegan (Oksanen et al., 2020).
Results
Patterns in water quality
There were both seasonal and spatial variations in water quality variables in the study area (Figures 3, 4). For most sites, DOC, TDN, TSS and TP were higher during the wet season compared with the dry season when TN, electrical conductivity and temperature were higher (Figure 3). At AGR and GRAS sites, POM reduced during the wet season indicating input of sediments poor in organic matter.
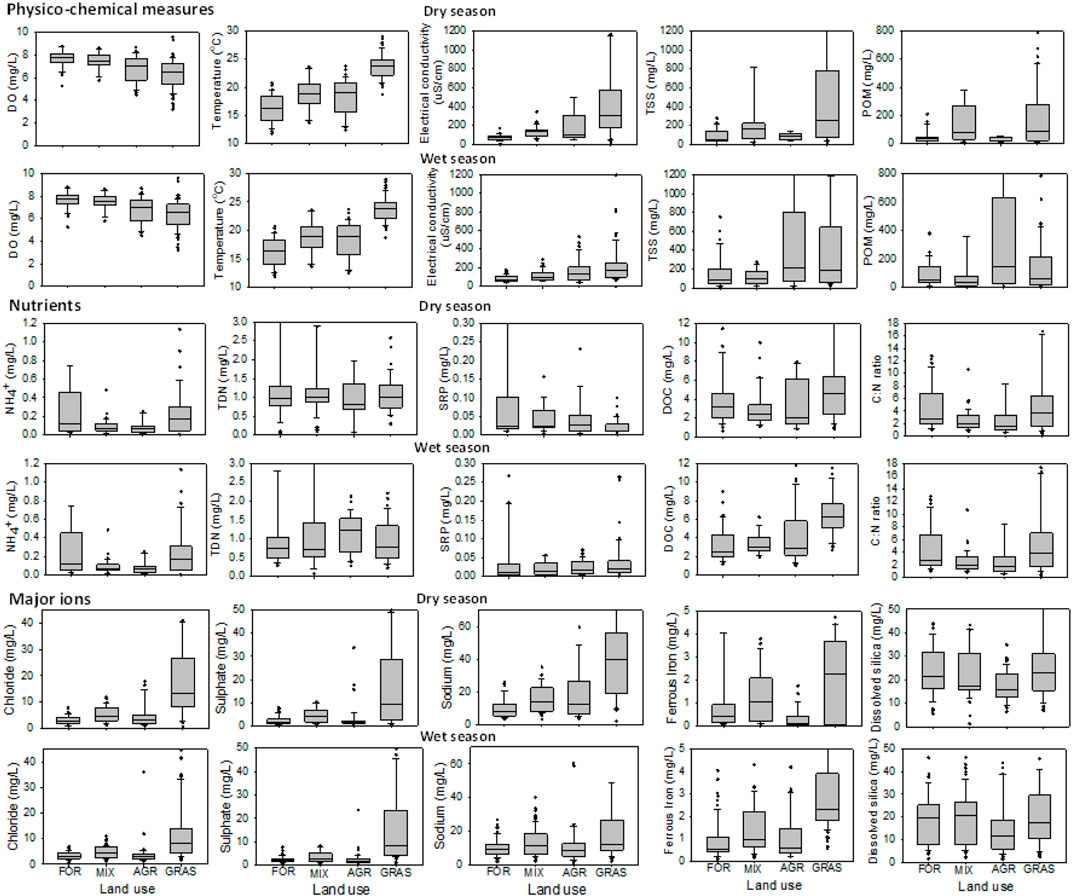
FIGURE 3. Box-and-whisker plots of water quality measures across different land use types in the Mara River basin during the dry and wet seasons. FOR = Forest, AGR = Agriculture, MIX = Mixed and GRAS = Grasslands.
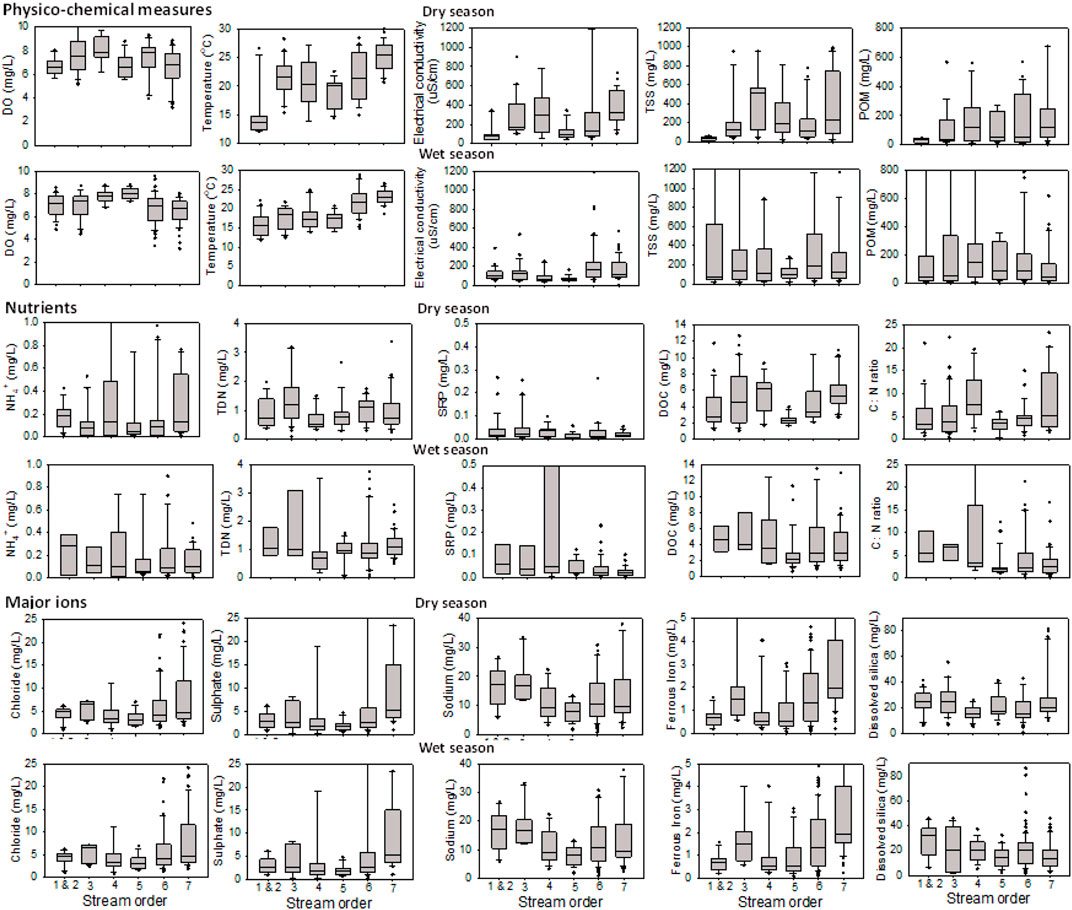
FIGURE 4. Box-and-whisker plots of water quality parameters across different stream orders (stream sizes) in the Mara River basin during the dry and wet seasons. FOR = Forest, AGR = Agriculture, MIX = Mixed and GRAS = Grasslands.
There were differences in physico-chemical measures, nutrients, and major ions among the land uses (Figure 3), but differences among stream orders were very minimal (Figure 4). There were significant differences in water quality variables between the dry and wet seasons (PERMANOVA F = 6.1, df = 1, p < 0.001), among land use types (PERMANOVA F = 12.3, df = 1, p < 0.001) but not among stream orders (PERMANOVA F = 1., df = 1, p = 0.096). There was a significant interaction of season with land use (PERMANOVA F = 2.6, df = 1, p = 0.026), but not of season with stream order (PERMANOVA F = 1.0, df = 1, p = 0.400). Neither was there an interaction between land use and stream order (PERMANOVA F = 1.4, df = 1, p = 0.198) nor among the three factors–season, land use and stream order (PERMANOVA F = 1.6, df = 1, p = 0.123). This implies that the influence of land use on water quality depended on the season. For variables with significant differences among land uses, GRAS sites had the highest levels of all physico-chemical variables (except DO concentrations) and concentrations of major ions (Figure 3). Although there were seasonal differences in water quality, trends among land use types (Figure 3) and stream orders (Figure 4) were the same during the dry and wet seasons.
Ordinations of water quality variables
In the PCA ordinations all the PCAs were statistically significant (PERMANOVA F = 3.8, df = 7, p = 012). We used three PCA axes (PC1-3) to explain the variance in water quality in the dataset. The first PCA axis (PC 1) accounted for the highest variance in the dataset with 38.1% while PC 2 and PC 3 accounted for 14.3% and 10.7% of the variance, respectively (Figure 5). The PC 1 is considered to be the land use axis or gradient along which sites were separated according to catchment land use with higher levels of electrical conductivity, water temperature and major ions (Cl−, K+, Na+, Ca2+ and Mg2+) in AGR and GRAS sites (Figure 5A). FOR sites recorded the highest DO levels and GRAS the lowest levels.
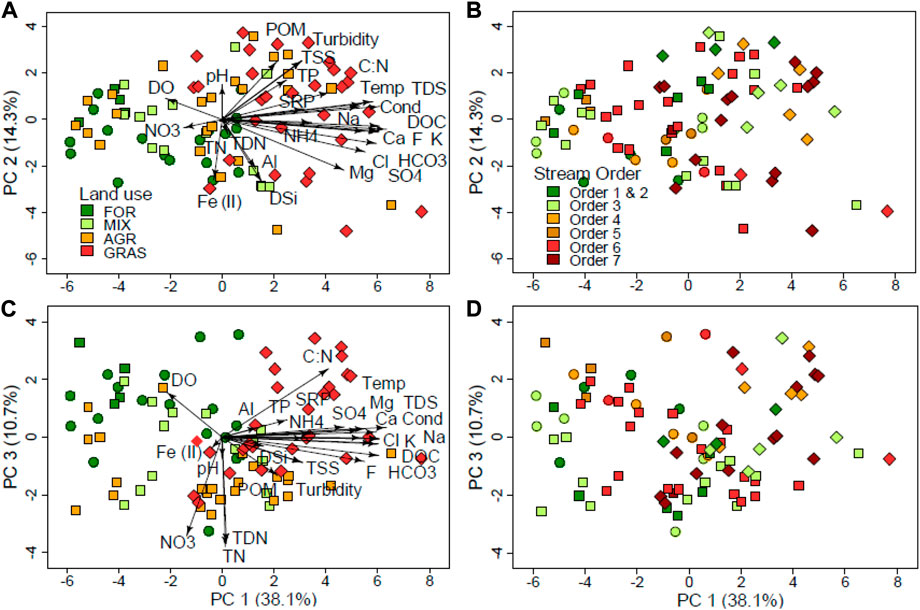
FIGURE 5. PCA biplot for water physico-chemistry variables across five land uses (regions) in the Mara River basin. The left panel (A,C) displays loadings for regions, and the right panel (B,D) shows loadings for stream size (stream orders). DO = dissolved oxygen, Temp = temperature, Cond = electrical conductivity, HCO3 = bicarbonate, NO3 = nitrates, SO4 = sulphate, DSi = dissolved silica.
The PC 2 separated sites according to turbidity, TSS, POM and TP with higher levels in AGR and GRAS (Figure 5A). However, the separation of water quality was not complete along PC 2, implying that despite the catchment land use influences, local reach-scale conditions also influenced some water quality characteristics. For instance, sites in the semi-arid areas of the MRB (GRAS sites) in the Olare-Orok and Talek tributaries and sites in the Mara River mainstem recorded high levels of electrical conductivity, water temperature and concentrations of major ions, including conservative ions (Cl−, F−,
The PC 3 was a nutrients gradient that separated sites according to nutrient concentrations. Surprisingly, AGR sites and GRAS sites were separated in terms of nutrient concentrations, whereby sites with high densities of livestock and wildlife (GRAS sites) had low concentrations of total and dissolved N (except ammonium) compared with AGR sites, but high concentrations of NH4+, SRP and TP concentrations (Figure 5C). Ammonia behaved differently compared to other species of N with high concentrations in GRAS and some AGR sites. The GRAS sites also had high C: N ratios, indicating a predominance of C compared to N in the water.
For all the three ordinations of physico-chemical variables, nutrients and major ions, stream order did not play a major role in the separation of the sites (Figures 5B,D). The only notable separation was on water chemistry (major ions) where stream orders 6 and 7 seemed separated from the rest of the smaller stream orders (Figures 3B,D). However, this is likely coincidental as most of the large river sections of the Mara River are also in semi-arid lowlands where other local influences are potentially playing a major role in influencing water chemistry and quality, such as high temperature due to aridity/increased evaporation, the influence of the large populations of livestock and large wildlife and potential changes in geology and erosion potential of the soils.
Drivers of water quality
A total of 20 model comparisons were made on the effects of different drivers on water quality variables in the Mara River (Supplementary Information). The best model identified for the GLMM model included four drivers without interactions - seasons, land use, stream order and the proportion of agricultural land in the catchments of the sampling sites (Percent AGR) (Tables 1). The model included the year of sampling and sampling site (nested within years) as random effects.
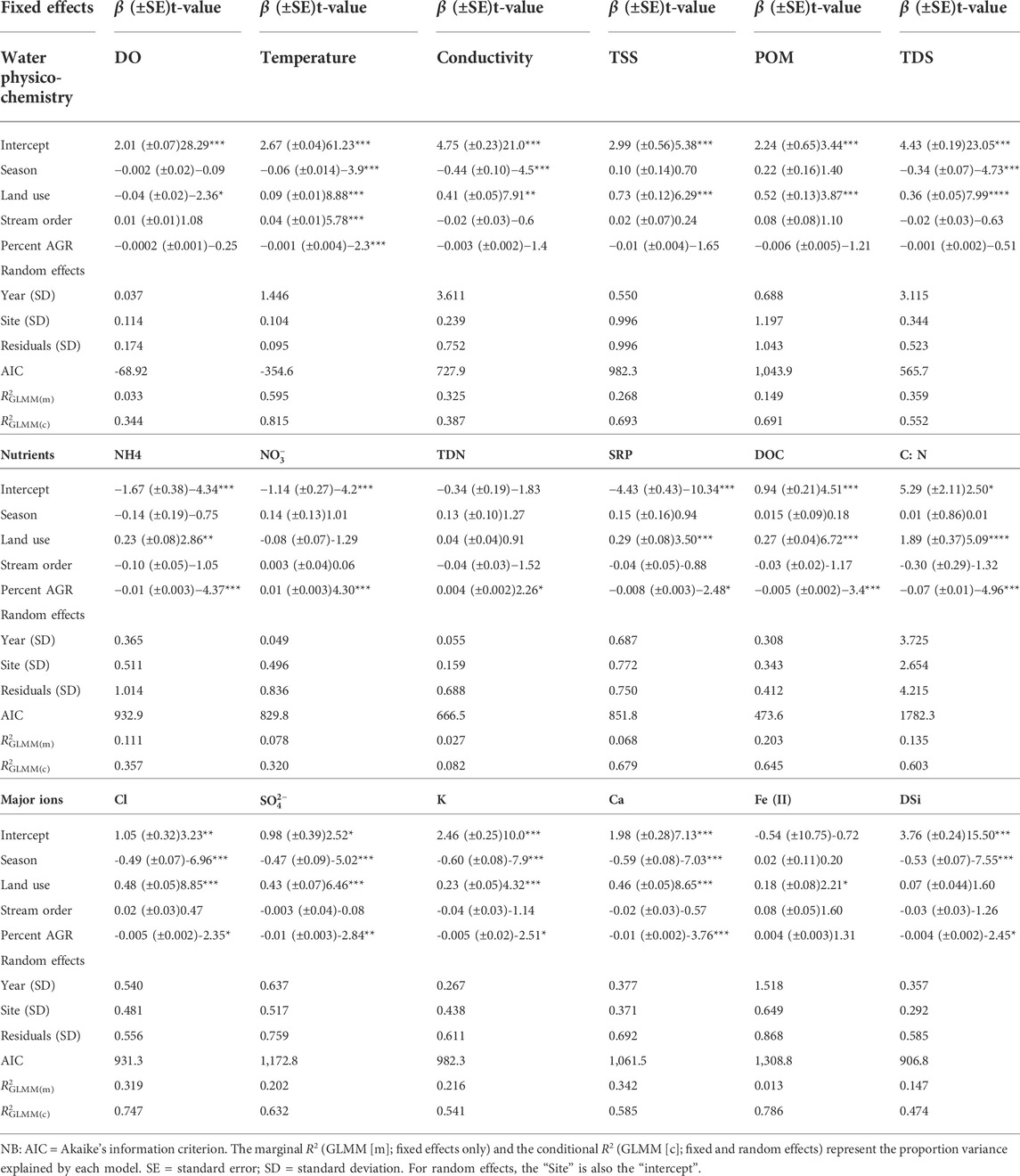
TABLE 1. Results of generalized linear mixed models (GLMM) of the effects of season, land use, stream order and percent agricultural land use in the catchments upstream of each sampling site (Percent AGR) on the physico-chemical water quality parameters, nutrients and major ions in the Mara River, Kenya. Physico-chemical measures included dissolved oxygen concentration (DO, mg/L), temperature (0C), electrical conductivity (µS/cm), total suspended solids (TSS, mg/L), particulate organic matter (POM, mg/L), and total dissolved solids (TDS, mg/L). Nutrients included ammonium (NH4, mg/L), nitrates (
The GLMM identified different responses in physico-chemical measures, nutrients and major ions to seasonality, land use, stream size and percent agricultural land use in the catchments of the sampling sites (Percent AGR) (Table 1). After a series of step-wise evaluation of many GLMM models, land use was identified as the main driver of water quality, followed by Percent AGR, seasonality then stream size. Land use had the strongest influence on water quality by influencing all (eighteen) variables except three (
Relationships among physico-chemical measures
Several relationships were explored between catchment characteristics and selected physico-chemical water quality variables (Figure 6). The regression converting TSS to turbidity has a slope of 1.051 and an R2 of 0.98 (Figure 6A), while that for POM has a factor of 0.157 and an R2 of 0.31 (Figure 6B). The low slope for the relationship between TSS and POM indicates that the proportion of POM in TSS (only 16%) was very low, an indication of large proportions of inorganic particles in TSS. Moreover, the relationship also shows that in large rivers the relationship is non-linear, indicating that small streams have higher amounts of organic matter, while large rivers transport more inorganic materials. The relationship between RDS and the proportion of grasslands in the catchments of the sampling sites (Percent GRAS) was positive (Figure 6C; r = 0.66, p < 0.001), which is expected given that the Mara River drains large areas of protected semi-arid areas in the MMNR, and conservancies used for livestock grazing.
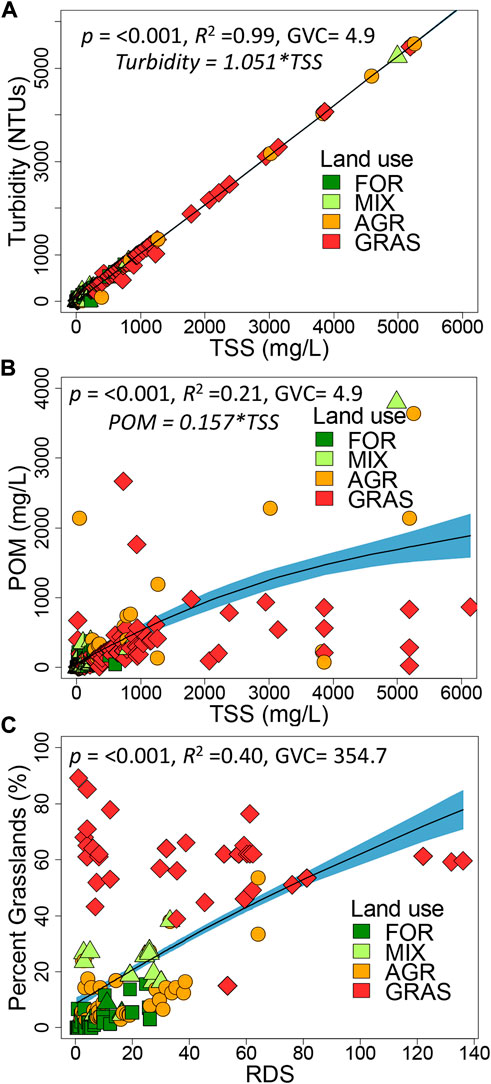
FIGURE 6. Relationships between different characteristics and water quality variables in the Mara River and its basin as explored using generalized additive models (GAMs). The relationships are between (A) TSS [mg/l] and turbidity [uS/cm], (B) TSS [mg/L] and POM [mg/L], and (C) RDS and Percent GRAS. To test the significance of the relationships, we fitted a GAM model with a smoothing function. The black line with light-blue shaded area represents smoother mean and s. e.; smoother significance, R2 and GCV are supplied in the figures.
Longitudinal trends in water quality variables
River distance from the source (RDS), and effectively stream size, had significant influences on many of the water quality variables as explored by GAMs (Figure 7). The stream size influenced dissolved oxygen and water temperature whereby DO concentration decreased with stream size, while water temperature increased as expected (Figure 7). Electrical conductivity had higher values as the distance increased from the sources (Figure 7). Interestingly, TSS and POM did not respond to changes in stream size, implying that other factors played a predominant role. Similarly, none of the nutrients responded to changes in stream size. Small agricultural streams and large grassland streams had high nutrient concentrations which diminished the influence of stream size on nutrient concentrations.
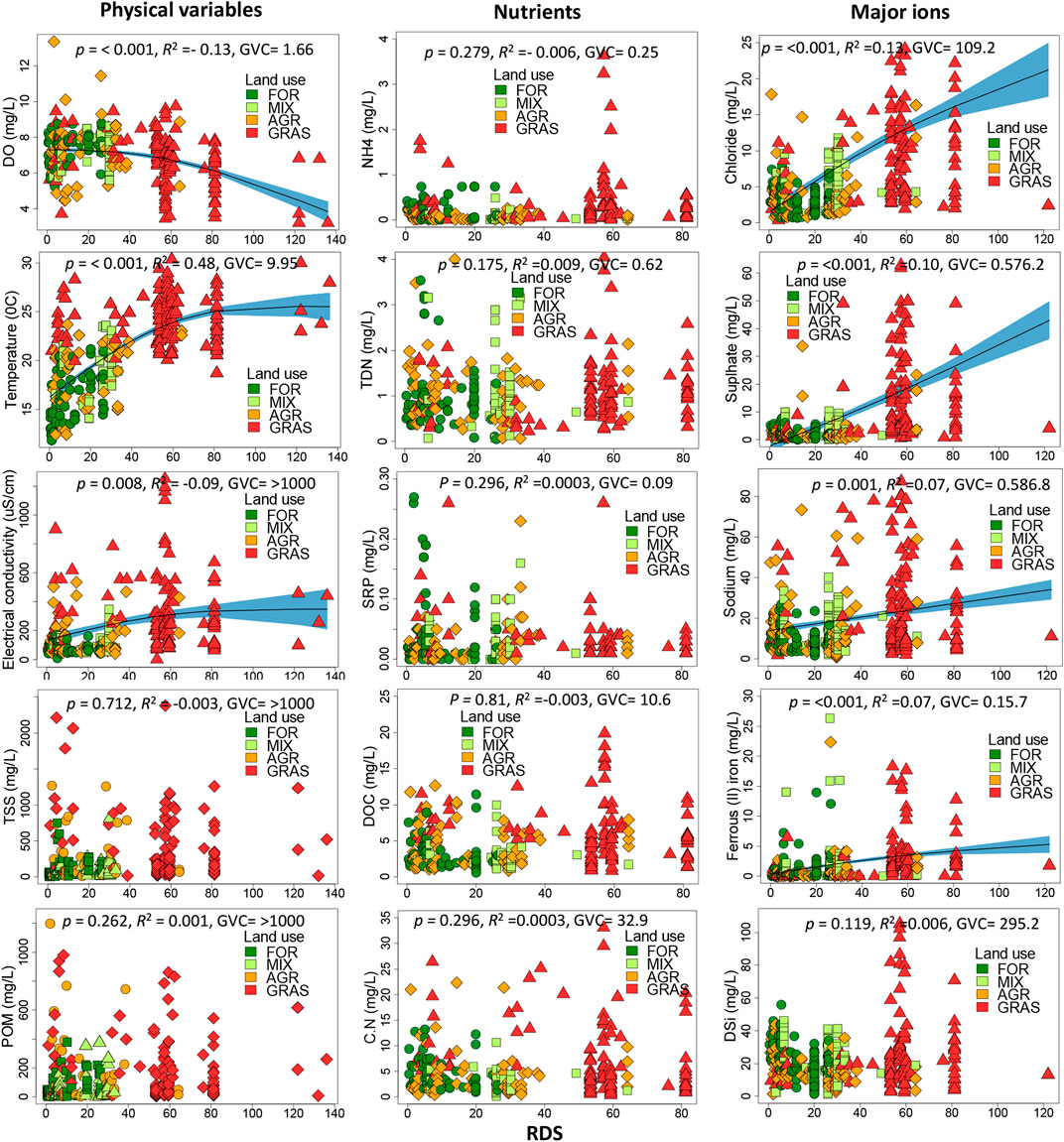
FIGURE 7. Longitudinal trends (changes with stream size) in water quality variables in the Mara River, Kenya as explored using generalized additive models (GAMs). RDS = river distance from source, determined as the square-root of catchment area in km2. To test the significance of the relationships, we fitted a GAM model with a smoothing function. The black line with light-blue shaded area represents smoother mean and s. e.; smoother significance, R2 and GCV are supplied in the figures that show significant relationships.
Major ions showed the strongest positive response to increasing stream size. Four solutes Cl−,
Discussion
In this study, we explored the influence of land use, seasonality and stream size or order as drivers of water quality in the Mara River using a dataset collected from 2010 to 2018. The results show that land use has the greatest influence on water quality and diminishes the influence of stream size as a predictor of water quality in the rivers. Specifically, agriculture and grazing were identified as the main drivers of water quality in the river with suspended sediments, turbidity, nutrients, and major ions (including conservative ions) having the highest concentrations in AGR and GRAS sites compared with FOR and MIX sites. Although seasonality played a major role in influencing water quality in the rivers, this influence is a likely amplification of land use effects through runoff, erosion and leaching of solutes. Despite the predicted influence of stream size on some physical and chemical characteristics of rivers, including different fractions of organic matter (Vannote et al., 1980), the influence of stream order on water quality was rather small in this study.
Influence of land use on water quality
The AGR and GRAS sites had lower levels of dissolved oxygen (DO) concentration, and higher levels of electrical conductivity, dissolved organic carbon and the ratio of C: N. These patterns have previously been reported in other Afrotropical rivers (Kroese et al., 2020; Dalu et al., 2022), but limited comparative data exist for Afromontane-savanna rivers. Specifically, the influence of land use and livestock grazing on water quality in rivers in semi-arid landscapes has been done anecdotally without basin-wide comparisons. This is of significance because agricultural land use and other human activities are likely to intensify as human populations in many African countries (averaging 2–3% p. a.) continue to grow. The most notable development over the years has been an expansion of agriculture and human settlements to marginal (semi-arid) areas of the basin; while natural forests reduced significantly between 1986 and 2000, grasslands reduced even at a higher rate (Serneels et al., 2001; Mati et al., 2008).
The land use influence on water quality was clearly expressed in total suspended sediments, turbidity, electrical conductivity, nutrients and major ions (including conservative ions), which were higher in AGR, and GRAS sites compared with FOR and MIX sites. These findings indicate that these water quality variables, especially electrical conductivity and major ions, respond to changes in catchment biogeochemical processes, such as runoff, mineralization and leaching of elements, that are brought about by land use change (Neill et al., 2006). Previous studies in Afrotropical rivers have also reported higher values of these measures for agricultural streams (Kibichii et al., 2007; Kasangaki et al., 2008).
Although AGR and GRAS sites had high concentrations of nutrients, the specific constituents differed between the two land uses. AGR sites had high amounts of
Conversion of native forest cover to farmlands is also accompanied by changes in the composition of vegetation in the riparian zones of streams and rivers. One such common change in highland streams in Kenya is plantation forestry of exotic tree species, which has significant negative effects on aquatic ecosystems (Reinhart and VandeVoort, 2006). Most riparian zones of agricultural streams are often lined with non-native trees, such as Eucalyptus, which have been linked to changes in organic matter standing stocks, modified food webs and flow regimes, reduced leaf processing by invertebrates and changes in the composition of aquatic communities in streams (Cooper et al., 2013; Masese et al., 2014a; Silva-Junior et al., 2014). Alteration of riparian vegetation can also influence water quality and nutrient cycling in streams. For instance, the clearance of riparian vegetation can increase mean water temperature in streams and lead to the entry of sediments and nutrients from agricultural lands through runoff (Minaya et al., 2013; Fugère et al., 2018; Kroese et al., 2020).
The high concentrations of ammonia and phosphorous in GRAS sites are likely linked to the high numbers of livestock and large wildlife in these areas that deposit large amounts of organic matter and nutrients in water through egestion and excretion during watering or crossings (Kanga et al., 2011; Subalusky et al., 2015; Iteba et al., 2021). That ammonia behaved differently from the other N species suggests that other factors were involved in its occurrence in the AGR sites. Previous studies in the river reported low DO levels at sites inhabited by hippos, especially during the dry season when an accumulation of organic matter in pools can drive DO to hypoxic or anoxic levels, which leads to the accumulation of ammonia from hippo excretion (Dutton et al., 2018; Dutton et al., 2021). High amounts of ammonia in some of the GRAS sites influenced by livestock are also linked to excretion in water by the livestock during watering and crossings (Iteba et al., 2021). Livestock access to stream can mobilize sediments leading to increased levels of turbidity, and their excretion and egestion in water can increase nutrients and organic matter.
Both AGR and GRAS sites recorded high levels of turbidity and TSS. An increase in turbidity or TSS is one of the most reported negative influences of livestock on water quality (Meehan and Platts, 1978; Belsky et al., 1999; Kroese et al., 2020). The GRAS sites are in savanna grasslands that host high populations of livestock, which can mobilize soils and render them susceptible to erosion. Previous studies on erosion have shown that grazing lands generate the highest sediment yield (Dunne, 1979), and increased erosion in the Talek catchment has previously been attributed to cattle grazing (Glover and Wateridge, 1968). Similarly, large numbers of wildlife, especially hippos that spend 50% of their time in the river (Subalusky et al., 2015), can mobilize sediments through bioturbation, impacting downstream river reaches (Dutton et al., 2018; Iteba et al., 2021).
Compared to AGR sites GRAS sites had higher levels of POM, DOC and carbon to nitrogen ratio (C: N) (Figure 5). An increase in POM relative to TSS in GRAS sites is interesting. It points to the input of large amounts of organic matter by livestock and hippos, which can increase the levels of C in the water column relative to other suspended materials. A previous study has shown that CPOM in the Mara River is mainly composed of hippo faeces (Subalusky et al., 2017). Similarly, livestock and hippo subsidies in the form of dung are very high in C relative to N and P (Subalusky et al., 2015; Iteba et al., 2021; Masese et al., 2022). During decomposition, hippo and cattle dung can be major sources of C in water which would elevate the amount of DOC and C in water relative to N and P (Masese et al., 2018; Subalusky et al., 2018). In the Mara River, studies have shown that hippo egestion accounts for 88% of total carbon (C) inputs from hippos (Subalusky et al., 2018), and such levels of inputs can explain the high C to N ratio at GRAS sites.
Despite the overwhelming influence of land use on water quality in streams and rivers in our study area, there is evidence that some of the dissolved constituents (especially the major ions) are likely driven by climate and geology. While the concentrations of biogenic solutes that are involved in biological processes (e.g., nutrients and carbon) are largely driven by land use (or land cover) and anthropogenic activities (Worrall and Burt, 2007; Van Meter et al., 2018; Kim et al., 2020), the concentrations of elements released from rock weathering (e.g., the major ions in this study) are thought to be driven by geology (Gaillardet et al., 1999; Ibarra et al., 2016). GRAS sites differ from the rest of the sites in terms of climate (the area is largely semi-arid with seasonal flows) and geology, and this can partly explain the elevated levels of solutes in these sites. The humid uplands in the Nyangores and Amala sub-catchments are underlain by quaternary volcanic deposits and Cambisols, while tertiary deposits and Vertisols dominate in the semi-arid lowlands (Sombroek et al., 1982; Jackson and McCarter, 1994). In addition to these differences, grassland streams are also characterized by reduced canopy cover, which, together with the semi-arid conditions and high evaporation rates, can concentrate solutes leading to elevated levels of major ions in streams, especially during the dry season.
The role of seasonality
Considering the wide variability in climatic conditions across the MRB and similar Afromontane-savanna rivers, seasonality is bound to play a major role in determining water quality and availability, especially during the dry season. Apart from differences in discharge levels that can influence solute concentrations, seasonality also influences the interaction with and use of streams and rivers by both people and their livestock. It has been noted that during the dry season, the dependence on streams and rivers as a source of water for domestic use and livestock watering is very high (Yillia et al., 2008). This increased traffic in rivers by people and livestock can have negative consequences on water quality. Previous studies have shown that seasonality can increase inter-site differences during the dry season due to a predominance of reach-scale influences (e.g., daily animal and human disturbance (Mathooko, 2001; Yillia et al., 2008; Minaya et al., 2013; Masese et al., 2014b). In contrast, during the wet season, rainfall events can make conditions in rivers uniform and increase longitudinal connectivity that reduces inter-site differences (Leung et al., 2012).
TSS, POM and DOC are some physico-chemical variables that showed strong responses to seasonality with higher concentrations during the wet season. This is linked to runoff and erosional processes that deliver large amounts of terrestrial organic matter and sediments from terrestrial to aquatic ecosystems. During the wet season, the strength of association between rivers and their catchments is increased when hydrologic flowpaths are activated, leading to increased delivery of solutes and organic matter, including sediments and nutrients, into rivers (Elsenbeer, 2001; Saunders et al., 2006). The amount of sediments and organic matter increased significantly in AGR and GRAS sites. This can be attributed to increased rates of sheet and gully erosion caused by livestock, bioturbation by hippos and loading of organic by both livestock and hippos (Glover & Wateridge, 1968; Dunne, 1979; Dutton et al., 2018).
It is expected that major ions, especially the elements driven by geology (Gaillardet et al., 1999; Ibarra et al., 2016), would show a geologic signal and have high concentrations during the dry season and low concentrations during the wet season because of dilution by rainwater. Some ions, such as Cl−,
The higher concentrations of Cl− and
Influence of stream size and longitudinal trends in water quality
The hypothesized influence of stream size or stream order on physico-chemical variables in the Mara River was not met. Only water temperature increased with an increase in stream size, as predicted from the opening of the canopy cover as rivers widen (Vannote et al., 1980) and the increase in ambient temperature occurring with lower elevation. The diminished influence of stream size on water quality was unexpected, but previous studies in this river have shown that land use change and human activities can alter biogeochemical processes in both low and high-order streams (Minaya et al., 2013; Mwanake et al., 2019). Similarly, reach-scale influences, such as watering of livestock, laundry washing and water abstractions by people and related disturbances abound along many rivers in the region leading to localized effects that can have a strong influence on water quality at the local scale (Mathooko, 2001; Yillia et al., 2008; Masese et al., 2009; Kroese et al., 2020).
Many attributes are predicted to have a linear or nonlinear response from low order to high order streams, e.g., water temperature, suspended sediments and the different fractions of organic matter (Vannote et al., 1980). However, in agreement with the diminished role of stream size on water quality variables in this study, several parameters did not respond to changes in stream size (Figure 4). As expected, there was a strong increase in water temperature, concomitant with a decrease in dissolved oxygen concentrations along the river (Figure 7). The electrical conductivity also increased downstream, probably linked to increased evaporation and accumulation of solutes in the semi-arid lower reaches of the river.
Expectedly, TSS and POM should increase with stream size because of the cumulative effects as low-order streams join to form large rivers. However, erosion from agricultural lands drained by the small streams and high livestock and wildlife (hippo) population in the lower reaches of the rivers can explain a lack of longitudinal trends in these two parameters, especially for TSS, as high levels were reported along the entire size gradient of the rivers (Figure 7). Similarly, there were no longitudinal trends in the concentrations of nutrients along the river. Similar levels were in small streams in agricultural areas and large river sections influenced by livestock and wildlife (hippos). Thus, the longitudinal patterns in TSS and nutrients observed in this study were confounded by changes in catchment land use and by the activities of livestock and large wildlife (Mwanake et al., 2019; Masese et al., 2022).
Among the water quality variables considered in this study, major ions showed the greatest response to changes in stream size. These patterns are typical of many other parameters that respond to changes in stream order. In the Lake Victoria basin, temperature, CPOM, FPOM and algal standing stocks (both benthic and water column) change with stream size, although expected patterns have been obscured by human activities that have modified riparian vegetation and in-stream conditions in rivers (Masese and McClain 2012).
Conclusion
The negative impacts of agriculture or land use in general on water quality in streams and rivers are a key concern for the catchment restoration and management of rivers in many regions around the world. Studies have shown that the influence of agriculture on water quality in streams does not only depend on the proportion of agricultural land in the catchment area, but also on the size and use of the riparian zone (Minaya et al., 2013; Kadeka et al., 2021). Similarly, low concentrations of suspended sediments and nutrients have been reported in agricultural streams that have a well-protected riparian zone (Kroese et al., 2020). These findings suggest that factors operating both at the catchment scale (e.g., the proportion of agricultural or urban land use) and local scale (e.g., the width and length of the riparian zone and its use) all have a role to play in explaining the quality of water in rivers (Schwarzenbach et al., 2010; Michalak, 2016). For water quality improvement, management efforts should focus to improve the conditions at both the catchment and local scales.
Our findings underline the role of land use, and most importantly, agriculture and grasslands, as drivers of water quality in Afrotropical rivers. Potential differences in catchment characteristics and, in our case, aridity and use (crop farming vs. grazing) need to be addressed in future studies to better constrain the effect of different land use practices and intensities on water quality in rivers. The results of this study are useful for stream restoration and management because they highlight the differences in water quality arising from agriculture and grazing. Furthermore, the findings show that responses in water quality to land uses might differ between regions of the same catchment that differ in climatic conditions (e.g., humid vs. semi-arid conditions). Thus, improved water quality in Afromontane-savanna rivers can only be improved by crafting management plans according to a region’s specific climatic characteristics. In this regard, the potential role of climate and climate change on water chemistry and water quality in Afromontane-savanna needs to be explored, especially the linkage between discharge and water quality variables to better understand fluxes and other attributes of solute concentrations and transport in these rivers.
Data availability statement
The raw data supporting the conclusion of this article will be made available by the authors, without undue reservation.
Author contributions
EW, FM, GM, and GS: conceptualization, experimental design, sampling; EW and FM: data compilation and data analysis; EW: drafting and editing of the manuscript; All authors commented on the manuscript and approved it for publication.
Acknowledgments
We are grateful to many people who contributed to the generation and compilation of the dataset used in this study. Joshua Kimeli helped with data compilation while Lubanga Lunaligo and David Namwaya (University of Eldoret) assisted during the laboratory analysis of some of the samples at the University of Eldoret. Robertson Marindany and Mutai Kitur assisted during fieldwork. We appreciate the technical assistance offered by Fred Kruis, Ferdi Battes, Lyzette Robbemont, Frank Weigman and Berrend Lolkema during sample analyses at the IHE-Delft laboratory, the Netherlands. We are also grateful to Tobias Goldhammer, Sarah Krocker and Claudia Schmalsch for help during the analysis of water chemistry samples at IGB, Berlin, Germany.
Conflict of interest
The authors declare that the research was conducted in the absence of any commercial or financial relationships that could be construed as a potential conflict of interest.
Publisher’s note
All claims expressed in this article are solely those of the authors and do not necessarily represent those of their affiliated organizations, or those of the publisher, the editors and the reviewers. Any product that may be evaluated in this article, or claim that may be made by its manufacturer, is not guaranteed or endorsed by the publisher.
Supplementary material
The Supplementary Material for this article can be found online at: https://www.frontiersin.org/articles/10.3389/fenvs.2022.972153/full#supplementary-material
References
Abrantes, K. G., Barnett, A., Marwick, T. R., and Bouillon, S. (2013). Importance of terrestrial subsidies for estuarine food webs in contrasting East African catchments. Ecosphere 4 (1), 1–33. doi:10.1890/es12-00322.1
Aich, V., Liersch, S., Vetter, T., Huang, S., Tecklenburg, J., Hoffmann, P., et al. (2014). Comparing impacts of climate change on streamflow in four large African river basins. Hydrol. Earth Syst. Sci. 18 (4), 1305–1321. doi:10.5194/hess-18-1305-2014
Allan, J. D. (2004). Landscapes and riverscapes: The influence of land use on stream ecosystems. Annu. Rev. Ecol. Evol. Syst. 35, 257–284. doi:10.1146/annurev.ecolsys.35.120202.110122
Anderson, M. J., and Walsh, D. C. (2013). PERMANOVA, ANOSIM, and the mantel test in the face of heterogeneous dispersions: What null hypothesis are you testing? Ecol. Monogr. 83 (4), 557–574. doi:10.1890/12-2010.1
Aufdenkampe, A. K., Mayorga, E., Raymond, P. A., Melack, J. M., Doney, S. C., Alin, S. R., et al. (2011). Riverine coupling of biogeochemical cycles between land, oceans, and atmosphere. Front. Ecol. Environ. 9 (1), 53–60. doi:10.1890/100014
Barton, K., and Barton, M. K. (2015). Package ‘mumin. AvaliableAt: https://CRAN.R-project.org/package=MuMIn.
Battin, T. J., Luyssaert, S., Kaplan, L. A., Aufdenkampe, A. K., Richter, A., and Tranvik, L. (2009). The boundless carbon cycle. Nat. Geosci. 2, 598–600. doi:10.1038/ngeo618
Belsky, A. J., Matzke, A., and Uselman, S. (1999). Survey of livestock influences on stream and riparian ecosystems in the Western United States. J. Soil Water Conservation 54 (1), 419–431.
Bernot, M. J., Sobota, D. J., Hall, R. O., Mulholland, P. J., Dodds, W. K., Webster, J. R., et al. (2010). Inter-regional comparison of land-use effects on stream metabolism. Freshw. Biol. 55, 1874–1890. doi:10.1111/j.1365-2427.2010.02422.x
Blecker, S. W., McCulley, R. L., Chadwick, O. A., and Kelly, E. F. (2006). Biologic cycling of silica across a grassland bioclimosequence. Global Biogeochemical Cycles 20 (3). doi:10.1029/2006GB002690
Bouillon, S., Yambélé, A., Spencer, R. G. M., Gillikin, D. P., Hernes, P. J., Six, J., et al. (2012). Organic matter sources, fluxes and greenhouse gas exchange in the Oubangui River (Congo River basin). Biogeosciences 9, 2045–2062. doi:10.5194/bg-9-2045-2012
Brauns, M., Allen, D. C., Boëchat, I. G., Cross, W. F., Ferreira, V., Graeber, D., et al. (2022). A global synthesis of human impacts on the multifunctionality of streams and rivers. Glob. Change Biol. 28, 4783–4793. doi:10.1111/gcb.16210
Burnham, K. P., and Anderson, D. R. (2004). Multimodel inference. Sociol. methods & Res. 33 (2), 261–304. doi:10.1177/0049124104268644
Chang, H. (2008). Spatial analysis of water quality trends in the Han River basin, South Korea. Water Res. 42 (13), 3285–3304. doi:10.1016/j.watres.2008.04.006
Chen, J., and Lu, J. (2016). Effects of land use, topography and socio-economic factors on river water quality in a mountainous watershed with intensive agricultural production in East China. PloS One 9, e102714. doi:10.1371/journal.pone.0102714
Cole, J. J., Prairie, Y. T., Caraco, N. F., McDowell, W. H., Tranvik, L. J., Striegl, R. G., et al. (2007). Plumbing the global carbon cycle: Integrating inland waters into the terrestrial carbon budget. Ecosystems 10, 172–185. doi:10.1007/s10021-006-9013-8
Cooper, S. D., Lake, P. S., Sabater, S., Melack, J. M., and Sabo, J. L. (2013). The effects of land use changes on streams and rivers in mediterranean climates. Hydrobiologia 719, 383–425. doi:10.1007/s10750-012-1333-4
Creed, I. F., McKnight, D. M., Pellerin, B. A., Green, M. B., Bergamaschi, B. A., Aiken, G. R., et al. (2015). the river as a chemostat: Fresh perspectives on dissolved organic matter flowing down the river continuum. Can. J. Fish. Aquat. Sci. 72 (8), 1272–1285. doi:10.1139/cjfas-2014-0400
Dalu, T., Mwedzi, T., Wasserman, R. J., Madzivanzira, T. C., Nhiwatiwa, T., and Cuthbert, R. N. (2022). Land use effects on water quality, habitat, and macroinvertebrate and diatom communities in African highland streams. Sci. Total Environ. 846, 157346. doi:10.1016/j.scitotenv.2022.157346
Diamantini, E., Lutz, S. R., Mallucci, S., Majone, B., Merz, R., and Bellin, A. (2018). Driver detection of water quality trends in three large European river basins. Sci. Total Environ. 612, 49–62. doi:10.1016/j.scitotenv.2017.08.172
Downing, J. A., Cole, J. J., Duarte, C. M., Middelburg, J. J., Melack, J. M., Prairie, Y. T., et al. (2012). Global abundance and size distribution of streams and rivers. Iw 2, 229–236. doi:10.5268/iw-2.4.502
Dudgeon, D., Arthington, A. H., Gessner, M. O., Kawabata, Z. I., Knowler, D. J., Lévêque, C., et al. (2006). Freshwater biodiversity: Importance, threats, status and conservation challenges. Biol. Rev. 81 (2), 163–182. doi:10.1017/S1464793105006950
Dudgeon, D. (2010). Prospects for sustaining freshwater biodiversity in the 21st century: Linking ecosystem structure and function. Curr. Opin. Environ. Sustain. 2 (5-6), 422–430. doi:10.1016/j.cosust.2010.09.001
Dunne, T. (1979). Sediment yield and land use in tropical catchments. J. Hydrology 42 (3-4), 281–300. doi:10.1016/0022-1694(79)90052-0
Dutton, C. L., Subalusky, A. L., Anisfeld, S. C., Njoroge, L., Rosi, E. J., and Post, D. M. (2018). The influence of a semi-arid sub-catchment on suspended sediments in the Mara River, Kenya. PLoS ONE 13 (2), e0192828. doi:10.1371/journal.pone.0192828
Dutton, C. L., Subalusky, A. L., Hamilton, S. K., Bayer, E. C., Njoroge, L., Rosi, E. J., et al. (2021). Alternative biogeochemical states of river pools mediated by hippo use and flow variability. Ecosystems 24 (2), 284–300. doi:10.1007/s10021-020-00518-3
Elsenbeer, H. (2001). Hydrologic flowpaths in tropical rainforest soilscapes?a review. Hydrol. Process. 15 (10), 1751–1759. doi:10.1002/hyp.237
Englmaier, G. K., Hayes, D. S., Meulenbroek, P., Terefe, Y., Lakew, A., Tesfaye, G., et al. (2020). Longitudinal river zonation in the tropics: Examples of fish and caddisflies from the endorheic awash river, Ethiopia. Hydrobiologia 847, 4063–4090. doi:10.1007/s10750-020-04400-0
FAO (2019). World fertilizer trends and outlook to 2022. Rome, Italy: FAO. AvaliableAt: https://www.fao.org/3/ca6746en/CA6746EN.pdf.
Fierro, P., Bertrán, C., Tapia, J., Hauenstein, E., Peña-Cortés, F., Vergara, C., et al. (2017). Effects of local land-use on riparian vegetation, water quality, and the functional organization of macroinvertebrate assemblages. Sci. Total Environ. 609, 724–734. doi:10.1016/j.scitotenv.2017.07.197
Fugère, V., Jacobsen, D., Finestone, E. H., and Chapman, L. J. (2018). Ecosystem structure and function of Afrotropical streams with contrasting land use. Freshw. Biol. 63, 1498–1513. doi:10.1111/fwb.13178
Gaillardet, J., Dupré, B., Louvat, P., and Allegre, C. J. (1999). Global silicate weathering and CO2 consumption rates deduced from the chemistry of large rivers. Chem. Geol. 159 (1-4), 3–30. doi:10.1016/s0009-2541(99)00031-5
Glover, P. E., and Wateridge, L. E. D. (1968). Erosion terraces in the loita-aitong areas of Kenya masailand. Afr. J. Ecol. 6 (1), 125–129. doi:10.1111/j.1365-2028.1968.tb00909.x
Godsey, S. E., Hartmann, J., and Kirchner, J. W. (2019). Catchment chemostasis revisited: Water quality responds differently to variations in weather and climate. Hydrol. Process. 33, 3056–3069. doi:10.1002/hyp.13554
Gregory, K. J., and Walling, D. E. (1973). Drainage basin form and process: Geomorphological approach. London: Edward Arnold.
Guo, D., Lintern, A., Webb, J. A., Ryu, D., Bende-Michl, U., Liu, S., et al. (2020). A data-based predictive model for spatiotemporal variability in stream water quality. Hydrol. Earth Syst. Sci. 24 (2), 827–847. doi:10.5194/hess-24-827-2020
Guo, D., Lintern, A., Webb, J. A., Ryu, D., Liu, S., Bende‐Michl, U., et al. (2019). Key factors affecting temporal variability in stream water quality. Water Resour. Res. 55, 112–129. doi:10.1029/2018wr023370
Hastie, T. J., and Tibshirani, R. J. (1990). Generalized additive models. New York, NY, USA: Chapman & Hall.
Hilary, B., Chris, B., North, B. E., Angelica Maria, A. Z. A., Sandra Lucia, A. Z. S., Carlos Alberto, Q. G. C., et al. (2021). Riparian buffer length is more influential than width on river water quality: A case study in southern Costa Rica. J. Environ. Manag. 286, 112132. doi:10.1016/j.jenvman.2021.112132
Hwang, S. A., Hwang, S. J., Park, S. R., and Lee, S. W. (2016). Examining the relationships between watershed urban land use and stream water quality using linear and generalized additive models. Water 8 (4), 155. doi:10.3390/w8040155
Hynes, H. B. N. (1975). The stream and its valley. SIL Proc. 1922-2010 19, 1–15. doi:10.1080/03680770.1974.11896033
Ibarra, D. E., Caves, J. K., Moon, S., Thomas, D. L., Hartmann, J., Chamberlain, C. P., et al. (2016). Differential weathering of basaltic and granitic catchments from concentration-discharge relationships. Geochimica Cosmochimica Acta 190, 265–293. doi:10.1016/j.gca.2016.07.006
Iteba, J. O., Hein, T., Singer, G. A., and Masese, F. O. (2021). Livestock as vectors of organic matter and nutrient loading in aquatic ecosystems in African savannas. PLoS ONE 16 (9), e0257076. doi:10.1371/journal.pone.0257076
Jacobs, S. R., Breuer, L., Butterbach-Bahl, K., Pelster, D. E., and Rufino, M. C. (2017). Land use affects total dissolved nitrogen and nitrate concentrations in tropical montane streams in Kenya. Sci. Total Environ. 603-604, 519–532. doi:10.1016/j.scitotenv.2017.06.100
Kadeka, E. C., Masese, F. O., Lusega, D. M., Sitati, A., Kondowe, B. N., and Chirwa, E. R. (2021). No difference in instream decomposition among upland agricultural and forested streams in Kenya. Front. Environ. Sci. 9, 794525. doi:10.3389/fenvs.2021.794525
Kanga, E. M., Ogutu, J. O., Olff, H., and Santema, P. (2011). Population trend and distribution of the Vulnerable common hippopotamus Hippopotamus amphibius in the Mara Region of Kenya. Oryx 45 (1), 20–27. doi:10.1017/S0030605310000931
Karr, J. R. (1998). “Rivers as sentinels: Using the biology of rivers to guide landscape management,” in River ecology and management: Lessons from the pacific coastal ecoregion. Editors R. J. Naiman, and R. E. Bilby (New York: Springer-Verlag), 502–528. doi:10.1007/978-1-4612-1652-0_20
Kasangaki, A., Chapman, L. J., and Balirwa, J. (2008). Land use and the ecology of benthic macroinvertebrate assemblages of high-altitude rainforest streams in Uganda. Freshw. Biol. 53, 681–697. doi:10.1111/j.1365-2427.2007.01925.x
Kibichii, S., Shivoga, W. A., Muchiri, M., and Miller, S. N. (2007). Macroinvertebrate assemblages along a land‐use gradient in the upper River Njoro watershed of Lake Nakuru drainage basin, Kenya. Lakes & Reserv. 12 (2), 107–117. doi:10.1111/j.1440-1770.2007.00323.x
Kilonzo, F., Masese, F. O., Van Griensven, A., Bauwens, W., Obando, J., and Lens, P. N. L. (2014). Spatial-temporal variability in water quality and macro-invertebrate assemblages in the Upper Mara River basin, Kenya. Phys. Chem. Earth, Parts A/B/C 67-69, 93–104. doi:10.1016/j.pce.2013.10.006
Kim, K. B., Jung, M. K., Tsang, Y. F., and Kwon, H. H. (2020). Stochastic modeling of chlorophyll-a for probabilistic assessment and monitoring of algae blooms in the Lower Nakdong River, South Korea. J. Hazard. Mater. 400, 123066. doi:10.1016/j.jhazmat.2020.123066
Lambert, T., Pierson-Wickmann, A. C., Gruau, G., Thibault, J. N., and Jaffrezic, A. (2011). Carbon isotopes as tracers of dissolved organic carbon sources and water pathways in headwater catchments. J. Hydrology 402, 228–238. doi:10.1016/j.jhydrol.2011.03.014
Lamberti, G. A., Chaloner, D. T., and Hershey, A. E. (2010). Linkages among aquatic ecosystems. J. North Am. Benthol. Soc. 29 (1), 245–263. doi:10.1899/08-166.1
Lenat, D. R., and Crawford, J. K. (1994). Effects of land use on water quality and aquatic biota of three North Carolina Piedmont streams. Hydrobiologia 294, 185–199. doi:10.1007/bf00021291
Leung, A. S. L., Li, A. O. Y., and Dudgeon, D. (2012). Scales of spatiotemporal variation in macroinvertebrate assemblage structure in monsoonal streams: The importance of season. Freshw. Biol. 57, 218–231. doi:10.1111/j.1365-2427.2011.02707.x
Li, L., Stewart, B., Zhi, W., Sadayappan, K., Ramesh, S., Kerins, D., et al. (2022). Climate controls on river chemistry. Earth's Future 10, e2021EF002603. doi:10.1029/2021EF002603
Lintern, A., Webb, J. A., Ryu, D., Liu, S., Bende‐Michl, U., Waters, D., et al. (2018). Key factors influencing differences in stream water quality across space. WIREs Water 5 (1), e1260. doi:10.1002/wat2.1260
Lovett, J. C., and Wasser, S. K. (1993). Biogeography and ecology of the rain forestsof eastern Africa. Cambridge: Cambridge University Press.
Maghanga, J. K., Kituyi, J. L., Kisinyo, P. O., and Ng’etich, W. K. (2013). Impact of nitrogen fertilizer applications on surface water nitrate levels within a Kenyan tea plantation. J. Chem. 2013, 1–4. doi:10.1155/2013/196516
Masese, F. O., Abrantes, K. G., Gettel, G. M., Bouillon, S., Irvine, K., and McClain, M. E. (2015). Are large herbivores vectors of terrestrial subsidies for riverine food webs? Ecosystems 18 (4), 686–706. doi:10.1007/s10021-015-9859-8
Masese, F. O., Abrantes, K. G., Gettel, G. M., Irvine, S., Bouillon, K., and McClain, M. E. (2018). Trophic structure of an african savanna river and organic matter inputs by large terrestrial herbivores: A stable isotope approach. Freshw. Biol. 63, 1365–1380. doi:10.1111/fwb.13163
Masese, F. O., Fuss, T., Bistarelli, L. T., Buchen-Tschiskale, C., and Singer, G. (2022). Large herbivorous wildlife and livestock differentially influence the relative importance of different sources of energy for riverine food webs. Sci. Total Environ. 828, 154452. doi:10.1016/j.scitotenv.2022.154452
Masese, F. O., Kitaka, N., Kipkemboi, J., Gettel, G. M., Irvine, K., and McClain, M. E. (2014b). Litter processing and shredder distribution as indicators of riparian and catchment influences on ecological health of tropical streams. Ecol. Indic. 46, 23–37. doi:10.1016/j.ecolind.2014.05.032
Masese, F. O., Kitaka, N., Kipkemboi, J., Gettel, G. M., Irvine, K., and McClain, M. E. (2014a). Macroinvertebrate functional feeding groups in Kenyan highland streams: Evidence for a diverse shredder guild. Freshw. Sci. 33 (2), 435–450. doi:10.1086/675681
Masese, F. O., and McClain, M. E. (2012). Trophic resources and emergent food web attributes in rivers of the Lake Victoria basin: A review with reference to anthropogenic influences. Ecohydrol. 5 (6), 685–707. doi:10.1002/eco.1285
Masese, F. O., Raburu, P. O., and Muchiri, M. (2009). A preliminary benthic macroinvertebrate index of biotic integrity (B-IBI) for monitoring the Moiben River, Lake Victoria Basin, Kenya. Afr. J. Aquatic Sci. 34 (1), 1–14. doi:10.2989/ajas.2009.34.1.1.726
Masese, F. O., Salcedo-Borda, J. S., Gettel, G. M., Irvine, K., and McClain, M. E. (2017). Influence of catchment land use and seasonality on dissolved organic matter composition and ecosystem metabolism in headwater streams of a Kenyan river. Biogeochemistry 132, 1–22. doi:10.1007/s10533-016-0269-6
Mathooko, J. M. (2001). Disturbance of a Kenya Rift Valley stream by the daily activitiesof local people and their livestock. Hydrobiologia 458, 131–139. doi:10.1023/a:1013160918203
Mati, B. M., Mutie, S., Gadain, H., Home, P., and Mtalo, F. (2008). Impacts of land‐use/cover changes on the hydrology of the transboundary Mara River, Kenya/Tanzania. Lakes & Reserv. 13, 169–177. doi:10.1111/j.1440-1770.2008.00367.x
Mazerolle, M. J. (2017). AICcmodavg: Model selection and multimodel inference based on (Q) AIC(c). AvaliableAt: https://CRAN.R-project.org/package=AICcmodavg.
McArdle, B. H., and Anderson, M. J. (2001). Fitting multivariate models to community data: A comment on distance-based redundancy analysis. Ecology 82 (1), 290–297. doi:10.1890/0012-9658(2001)082[0290:fmmtcd]2.0.co;2
McClain, M. E., Subalusky, A. L., Anderson, E. P., Dessu, S. B., Melesse, A. M., Ndomba, P. M., et al. (2014). Comparing flow regime, channel hydraulics, and biological communities to infer flow–ecology relationships in the Mara River of Kenya and Tanzania. Hydrological Sci. J. 59 (3-4), 801–819. doi:10.1080/02626667.2013.853121
Meehan, W. R., and Platts, W. S. (1978). Livestock grazing and the aquatic environment. J. Soil Water Conservation 33 (6), 274–278.
Mello, K., Valente, R. A., Randhir, T. O., dos Santos, A. C. A., and Vettorazzi, C. A. (2018). Effects of land use and land cover on water quality of low-order streams in Southeastern Brazil: Watershed versus riparian zone. Catena 167, 130–138. doi:10.1016/j.catena.2018.04.027
Meybeck, M. (1987). Global chemical weathering of surficial rocks estimated from river dissolved loads. Am. J. Sci. 287, 401–428. doi:10.2475/ajs.287.5.401
Michalak, A. M. (2016). Study role of climate change in extreme threats to water quality. Nature 535 (7612), 349–350. doi:10.1038/535349a
Minaya, V., McClain, M. E., Moog, O., Omengo, F., and Singer, G. (2013). Scale-dependent effects of rural activities on benthic macroinvertebrates and physico-chemical characteristics in headwater streams of the Mara River, Kenya. Ecol. Indic. 32, 116–122. doi:10.1016/j.ecolind.2013.03.011
Mokaya, S. K., Mathooko, J. M., and Leichtfried, M. (2004). Influence of anthropogenic activities on water quality of a tropical stream ecosystem. Afr. J. Ecol. 42, 281–288. doi:10.1111/j.1365-2028.2004.00521.x
Mushi, C. A., Ndomba, P. M., Trigg, M. A., Tshimanga, R. M., and Mtalo, F. (2019). Assessment of basin-scale soil erosion within the Congo river basin: A review. CATENA 178, 64–76. doi:10.1016/j.catena.2019.02.030
Mwanake, R. M., Gettel, G. M., Aho, K. S., Namwaya, D. W., Masese, F. O., Butterbach‐Bahl, K., et al. (2019). Land use, not stream order, controls N 2 O concentration and flux in the upper Mara River basin, Kenya. JGR Biogeosciences 124 (11), 3491–3506. doi:10.1029/2019JG005063
Nagy, R. C., Lockaby, B. G., Kalin, L., and Anderson, C. (2012). Effects of urbanization on stream hydrology and water quality: The Florida Gulf Coast. Hydrol. Process. 26, 2019–2030. doi:10.1002/hyp.8336
Neill, C., Deegan, L. A., Thomas, S. M., Haupert, C. L., Krusche, A. V., Ballester, V. M., et al. (2006). Deforestation alters the hydraulic and biogeochemical characteristics of small lowland Amazonian streams. Hydrol. Process. 20, 2563–2580. doi:10.1002/hyp.6216
Obati, G. O. (2007). An investigation of forest ecosystem health in relation to anthropogenic disturbance in the south-Western Mau forest reserve. Kenya: Universität Bremen. Doctoral dissertation.
Ogutu, J. O., Piepho, H.-P., Said, M. Y., Ojwang, G. O., Njino, L., Kifugo, S. C., et al. (2016). Extreme wildlife declines and concurrent increase in livestock numbers in Kenya: What are the causes? PLoS ONE 11, e0163249. doi:10.1371/journal.pone.0163249
Oksanen, J., Blanchet, F. G., Friendly, M., Kindt, R., Legendre, P., McGlinn, D., et al. (2020). vegan: Community Ecology Package. R package version 2.5-6. Available from: http://CRAN.R-project.org/package=vegan.
Paetzold, A., Sabo, J. L., Sadler, J. P., Findlay, S. E., and Tockner, K. (2008). “Aquatic–terrestrial subsidies along river corridors,” in Hydroecology and ecohydrology: Past, present and future. (Chichester, UK: John Wiley & Sons), 57–92.
Petrone, K. C. (2010). Catchment export of carbon, nitrogen, and phosphorus across an agro‐urban land use gradient, Swan‐Canning River system, southwestern Australia. J. Geophys. Res. Biogeosciences 115, 1051. doi:10.1029/2009jg001051
Pinheiro, J., Bates, D., DebRoy, S., Sarkar, D., Heisterkamp, S., Van Willigen, B., et al. (2017). Package ‘nlme Linear and nonlinear mixed effects models, version. AvaliableAt: https://CRAN.R-project.org/package=nlme.
Potter, P., Ramankutty, N., Bennett, E. M., and Donner, S. D. (2010). Characterizing the spatial patterns of global fertilizer application and manure production Earth Interact. Earth Interact. 14, 1–22. doi:10.1175/2009EI288.1
Putri, M. S. A., Lou, C. H., Syai’in, M., Ou, S. H., and Wang, Y. C. (2018). Long-term river water quality trends and pollution source apportionment in Taiwan. Water 10 (10), 1394. doi:10.3390/w10101394
R Core Team (2020). R: A language and environment for statistical computing. Vienna, Austria: R Foundation for Statistical Computing, 101–142. AvaliableAt: https://www.r-project.org/extensions in ecology with R.
Rasmussen, J. B., Trudeau, V., and Morinville, G. R. (2009). Estimating the scale of fish feeding movements in rivers using δ13Csignature gradients. J. Animal Ecol. 78, 674–685. doi:10.1111/j.1365-2656.2008.01511.x
Reinhart, K. O., and VandeVoort, R. (2006). Effect of native and exotic leaf litter on macroinvertebrate communities and decomposition in a western Montana stream. Divers. Distrib. 12 (6), 776–781. doi:10.1111/j.1472-4642.2006.00252.x
Roach, K. A., Winemiller, K. O., and Davis, S. E. (2014). Autochthonous production in shallow littoral zones of five floodplain rivers: Effects of flow, turbidity and nutrients. Freshw. Biol. 59 (6), 1278–1293. doi:10.1111/fwb.12347
Roberts, A. D., and Prince, S. D. (2010). Effects of urban and non-urban land cover on nitrogen and phosphorus runoff to Chesapeake Bay. Ecol. Indic. 10, 459–474. doi:10.1016/j.ecolind.2009.07.017
Rodrigues, V., Estrany, J., Ranzini, M., de Cicco, V., Martín-Benito, J. M. T., Hedo, J., et al. (2018). Effects of land use and seasonality on stream water quality in a small tropical catchment: The headwater of Córrego Água Limpa, São Paulo (Brazil). Sci. total Environ. 622-623, 1553–1561. doi:10.1016/j.scitotenv.2017.10.028
Sanderman, J., Lohse, K. A., Baldock, J. A., and Amundson, R. (2009). Linking soils and streams: Sources and chemistry of dissolved organic matter in a small coastal watershed. Water Resour. Res. 45, W034018. doi:10.1029/2008WR006977
Saunders, T. J., McClain, M. E., and Llerena, C. A. (2006). The biogeochemistry of dissolved nitrogen, phosphorus, and organic carbon along terrestrial-aquatic flowpaths of a montane headwater catchment in the Peruvian Amazon. Hydrol. Process. 20 (12), 2549–2562. doi:10.1002/hyp.6215
Schoelynck, J., Müller, F., Vandevenne, F., Bal, K., Barão, L., Smis, A., et al. (2014). Silicon-vegetation interaction in multiple ecosystems: A review. J. Veg. Sci. 25, 301–313. doi:10.1111/jvs.12055
Schwarzenbach, R. P., Egli, T., Hofstetter, T. B., Von Gunten, U., and Wehrli, B. (2010). Global water pollution and human health. Annu. Rev. Environ. Resour. 35 (1), 109–136. doi:10.1146/annurevenviron-100809-125342
Serneels, S., Said, M. Y., and Lambin, E. F. (2001). Land cover changes around a major east african wildlife reserve: The Mara ecosystem (Kenya). Int. J. Remote Sens. 22 (17), 3397–3420. doi:10.1080/01431160152609236
Silva-Junior, E. F., Moulton, T. P., Boëchat, I. G., and Gücker, B. (2014). Leaf decomposition and ecosystem metabolism as functional indicators of land use impacts on tropical streams. Ecol. Indic. 36, 195–204. doi:10.1016/j.ecolind.2013.07.027
Smart, J. S. (1972). Quantitative characterization of channel network structure. Water Resour. Res. 8, 1487–1496. doi:10.1029/WR008i006p01487
Stenfert Kroese, J. S., Jacobs, S. R., Tych, W., Breuer, L., Quinton, J. N., and Rufino, M. C. (2020). Tropical montane forest conversion is a critical driver for sediment supply in East African catchments. Water Resour. Res. 56, e2020WR027495. doi:10.1029/2020WR027495
Strahler, W. G., Braun, H. M. H., and Van Der Pouw, B. J. A. (1982). Quantitative analysis of watershed geomorphology. Trans. AGU 38 (6), 913–920. doi:10.1029/TR038i006p00913
Strahler, A. N. (1957). Quantitative analysis of watershed geomorphology. Eos, Transactions American Geophysical Union 38 (6), 913–920. doi:10.1029/TR038i006p00913
Strauch, A. M. (2012). Seasonal variability in faecal bacteria of semiarid rivers in the Serengeti National Park, Tanzania. Mar. Freshw. Res. 62 (10), 1191–1200. doi:10.1071/mf11075
Subalusky, A. L., Dutton, C. L., Njoroge, L., Post, E. J. D. M., and Post, D. M. (2018). Organic matter and nutrient inputs from large wildlife influence ecosystem function in the Mara River, Africa. Ecology 99 (11), 2558–2574. doi:10.1002/ecy.2509
Subalusky, A. L., Dutton, C. L., Rosi, E. J., and Post, D. M. (2017). Annual mass drownings of the Serengeti wildebeest migration influence nutrient cycling and storage in the Mara River. Proc. Natl. Acad. Sci. U.S.A. 114, 7647–7652. doi:10.1073/pnas.1614778114
Subalusky, A. L., Dutton, C. L., Rosi-Marshall, E. J., and Post, D. M. (2015). The hippopotamus conveyor belt: Vectors of carbon and nutrients from terrestrial grasslands to aquatic systems in sub-saharan Africa. Freshw. Biol. 60, 512–525. doi:10.1111/fwb.12474
Tamooh, F., Van den Meersche, K., Meysman, F., Marwick, T. R., Borges, A. V., Merckx, R., et al. (2012). Distribution and origin of suspended matter and organic carbon pools in the Tana River Basin, Kenya. Biogeosciences 9 (8), 2905–2920. doi:10.5194/bg-9-2905-2012
Tank, J. L., Rosi-Marshall, E. J., Griffiths, N. A., Entrekin, S. A., and Stephen, M. L. (2010). A review of allochthonous organic matter dynamics and metabolism in streams. J. North Am. Benthol. Soc. 29 (1), 118–146. doi:10.1899/08-170.1
Thompson, S. E., Basu, N. B., Lascurain, J., Aubeneau, A., and Rao, P. S. C. (2011). Relative dominance of hydrologic versus biogeochemical factors on solute export across impact gradients. Water Resour. Res. 47, W00J05. doi:10.1029/2010WR009605
Tong, S. T. Y., and Chen, W. (2002). Modeling the relationship between land use and surface water quality. J. Environ. Manag. 66, 377–393. doi:10.1006/jema.2002.0593
Vannote, R. L., Minshall, G. W., Cummins, K. W., Sedell, J. R., and Cushing, C. E. (1980). The river continuum concept. Can. J. Fish. Aquat. Sci. 37, 130–137. doi:10.1139/f80-017
Van Meter, K. J., Van Cappellen, P., and Basu, N. B. (2018). Legacy nitrogen may prevent achievement of water quality goals in the Gulf of Mexico. Science 360 (6387), 427–430. doi:10.1126/science.aar4462
Veldhuis, M. P., Ritchie, M. E., Ogutu, J. O., Morrison, T. A., Beale, C. M., Estes, A. B., et al. (2019). Cross-boundary human impacts compromise the Serengeti-Mara ecosystem. Science 363, 1424–1428. doi:10.1126/science.aav05610.1126/science.aav0564
Vörösmarty, C. J., McIntyre, P. B., Gessner, M. O., Dudgeon, D., Prusevich, A., Green, P., et al. (2010). Global threats to human water security and river biodiversity. Nature 467, 555–561. doi:10.1038/nature09440
Wang, X., Zhang, L., Zhao, Z., and Cai, Y. (2018). Heavy metal pollution in reservoirs in the hilly area of southern China: Distribution, source apportionment and health risk assessment. Sci. Total Environ. 634, 158–169. doi:10.1016/j.scitotenv.2018.03.340
Wass, P. (1995). Kenya's Indigenous Forests: Status, Management, and Conservation. Switzerland and Cambridge, UK: IUCN. Gland.
Worrall, F., and Burt, T. P. (2007). Flux of dissolved organic carbon from UK rivers. Global Biogeochem. Cycles 21 (1). doi:10.1029/2006GB002709
Williamson, C. E., Dodds, W., Kratz, T. K., and Palmer, M. A. (2008). Lakes and streams as sentinels of environmental change in terrestrial and atmospheric processes. Front. Ecol. Environ. 6 (5), 247–254. doi:10.1890/070140
Wood, S., and Wood, M. S. (2015). Package ‘mgcv’ R package version, AvaliableA: https://CRAN.R-project.org/package=mgcv. 1–7.
Woodward, G., Gessner, M. O., Giller, P. S., Gulis, V., Hladyz, S., Lecerf, A., et al. (2012). Continental-scale effects of nutrient pollution on stream ecosystem functioning. Science 336, 1438–1440. doi:10.1126/science.1219534
Yillia, P. T., Kreuzinger, N., and Mathooko, J. M. (2008). The effect of in-stream activities on the Njoro River, Kenya. Part I. Stream flow and chemical water quality. Phys. Chem. Earth 33, 22–28. doi:10.1016/j.pce.2008.06.041
Keywords: linear mixed models, land use, non-linear relationships, savanna rivers, afrotropical rivers, water quality
Citation: Wanderi EW, Gettel GM, Singer GA and Masese FO (2022) Drivers of water quality in Afromontane-savanna rivers. Front. Environ. Sci. 10:972153. doi: 10.3389/fenvs.2022.972153
Received: 17 June 2022; Accepted: 09 September 2022;
Published: 12 October 2022.
Edited by:
José Maria Santos, University of Lisbon, PortugalReviewed by:
Rui Manuel Vitor Cortes, University of Trás-os-Montes and Alto Douro, PortugalSiyue Li, Wuhan Institute of Technology, China
Copyright © 2022 Wanderi, Gettel, Singer and Masese. This is an open-access article distributed under the terms of the Creative Commons Attribution License (CC BY). The use, distribution or reproduction in other forums is permitted, provided the original author(s) and the copyright owner(s) are credited and that the original publication in this journal is cited, in accordance with accepted academic practice. No use, distribution or reproduction is permitted which does not comply with these terms.
*Correspondence: Elizabeth W. Wanderi, ewanderi428@gmail.com; Frank O. Masese, f.masese@gmail.com